목록Artificial Intelligence/Natural Language Processing (9)
Code&Data Insights
https://www.aitimes.com/news/articleView.html?idxno=158463 "2년 내 LLM 학습 데이터 고갈...데이터 문제로 AI 발전 중단될 것" - AI타임스급속도로 성장하는 인공지능(AI) 모델이 데이터 부족 문제로 위협받고 있다는 지적이 나왔다. 현재 인터넷상에 존재하는 데이터만으로는 2년 내 AI 모델 성능을 높이는 데 한계를 맞을 것이라는www.aitimes.com요약 : AI 모델인 GPT-4나 구글의 제미나이와 같은 기술은 데이터 부족에 직면하고 있음. 데이터 요구량은 증가했지만, 품질이 낮거나 저작권 문제로 인해 접근이 제한되어 있기 떄문!--> 이에 대안으로 합성 데이터를 사용할 수 있지만, 모델 붕괴 가능성이 있습니다. 이러한 이유로 향후에는 도메인별..
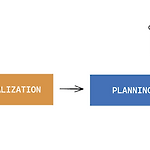
https://thegradient.pub/car-gpt/ Car-GPT: Could LLMs finally make self-driving cars happen?Exploring the utility of large language models in autonomous driving: Can they be trusted for self-driving cars, and what are the key challenges?thegradient.pub 최근 애플이 자율 주행 전기차 사업을 접었는데, 저를 포함하여 자율 주행 차의 미래에 대해 궁금함을 가진 분들에게 좋은 아티클이라고 생각해 공유하게 되었습니다. 초기 자율주행차의 구현 모델 이후,End-To-End learning을 도입했지만 여전히 a ..
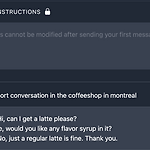
WITHOUT PROMPT => Prompt Instruction 없이 매우 평범하고 짧은 대화가 생성됨 WITH 1st PROMPT => 처음 대화보다 좀 더 친근한 버전이지만, 실생활에서 다른 대화 없이 주문을 받고 난 뒤 우리 커피샵을 골라줘서 고마워 이렇게 말하는 것은 어색하다고 생각함. WITH 2nd PROMPT => 원래 의도는 손님이 원하지 않는 경우에도 베이커리 메뉴 추천을 해서 수익을 올리려는 목적인데, 애초에 생성된 대화 내용이 손님이 추천을 원하는 경우로 나왔다. WITH 3rd PROMPT : 같은 prompt instruction이지만, prompt를 상세하게 설정(손님이 커피만 시키고 싶어하는 경우) => 의도한 결과와 실제상황과 가까운 자연스러운 대화가 생성됨!
Generative AI : 트레이닝 데이터를 기반으로 다양한 포맷(text, images, code, audio, video)의 새로운 컨텐츠를 생성해주는 AI 모델. Generative AI models are trained on substantial datasets of existing content and learn to generate new content similar to the data they were trained on. - Generative AI can start from the prompt, prompt can be Text/ Image/ Video/etc and then, it generates a new content as text/images/audio/video/code/d..
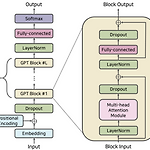
Large Language Model (LLM) : 자연어 처리를 위해 방대한 양의 데이터로 학습된 인공지능 모델로 인간과 유사한 텍스트를 처리, 이해 및 생성하도록 설계된 고급 AI 시스템. A type of deep learning model trained on massive amounts of text data to learn the patterns and structures of language. They can perform language-related tasks, including text generation, translation, summarization, sentiment analysis, and more. - LLMs work by training on diverse language ..
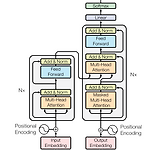
Attention - Contextual embedding => Transform each input embedding into a contextual imbedding => Model learns attention weights - Self-attention : allows to enhance the embedding of an input word by including information about its context - Encoder-Decoder Attention : Attention between words in the input sequence and words in the output sequence => how words from two sequences influence each ot..
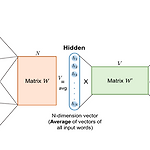
Word Embeddings · Word Vectors - Simple approach : one-hot vectors => NOT represent word meanining => Similarity/distance between all one hot vectors is the same => Better approach : ‘Word Embeddings’! · Word2Vec : how likely is a word w likely to show up near another word? - Extract the learned weights as the word embeddings - Use as training set, readily available texts, no need for hand-label..
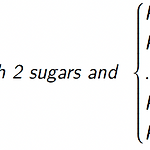
N-gram models : a probability distribution over sequence of events · Models the order of the events · Used when the past sequence of events is a good indicator of the next event to occur in the sequence -> To predict the next event in a sequence of event · Allows to compute the probability of the next item in a sequence · Or the probability of a complete sequence · Applications of Language Model..